MongoDB’s acquisition of Voyage AI for $220 million has sent ripples across the AI and database industry, sparking debates on whether this is a strategic masterstroke or just another case of AI-driven FOMO. The acquisition marks MongoDB’s push into AI-powered search and retrieval, but is it truly a game-changing integration, or is MongoDB simply playing catch-up in the AI race?
Is MongoDB Trying to Kill the Vector Database?
Prior to acquiring Voyage AI, MongoDB was already a full-featured vector database, allowing users to store and search operational data alongside vectors within a single system for intelligent search and generative AI applications. Unlike bolt-on vector solutions, its integrated vector search reduces infrastructure complexity, saving both time and cost.
Since its launch in 2023, MongoDB Atlas Vector Search has been one of the most widely used vector databases, according to the Retool State of AI report, with major enterprises like Novo Nordisk, Okta, and VISO TRUST leveraging its capabilities.
With Atlas Vector Search built into the core database, developers no longer need to sync data between operational and vector databases—eliminating complexity, reducing errors, and improving efficiency. While Voyage AI enhances embedding generation and query reranking, it does not handle large-scale vector storage and retrieval—a capability MongoDB already provides through Atlas Vector Search. Together, the two aim to offer a more seamless solution for AI-driven applications.
MongoDB’s stance? CEO Dev Ittycheria asserts that integrating Voyage AI makes “AI-powered search and retrieval native to the database,” removing the need for fragmented solutions. But the bigger question remains: Is MongoDB truly replacing dedicated vector databases, or is this just a well-crafted positioning strategy?
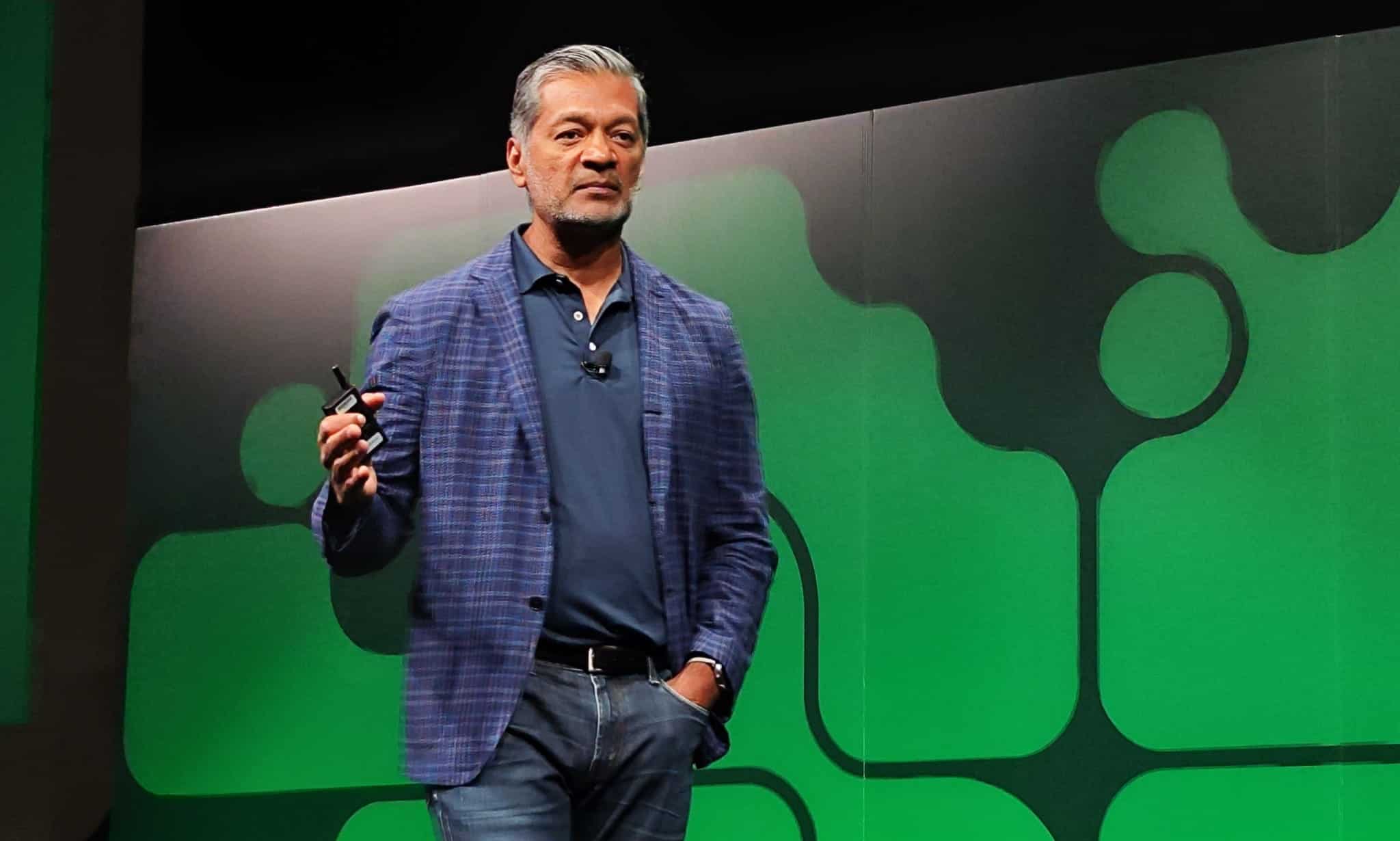
Did MongoDB Outmaneuver Databricks and Snowflake?
The acquisition has also raised eyebrows in the competitive AI database landscape, with Databricks and Snowflake reportedly in the race to acquire similar technology. MongoDB beat them to it, which might be seen as a win—but at what cost?
Snowflake, for instance, has been rapidly expanding its AI capabilities through acquisitions like Neeva, while Databricks acquired MosaicML to enhance its AI and ML ecosystem. MongoDB’s move suggests that instead of competing directly in the model-building space, it is betting on AI-native database integrations. The $220M price tag—roughly 2x Voyage AI’s last funding round valuation—suggests MongoDB was willing to pay a premium to stay relevant.
However, MongoDB’s real challenge is execution. While the acquisition provides a compelling AI narrative, integrating Voyage AI seamlessly into MongoDB Atlas while keeping up with the high-performance demands of vector search is easier said than done.
A Strategic Fit or an Overhyped Bet?
From a strategic standpoint, MongoDB’s move is not just about search—it’s about capturing the growing market for Retrieval-Augmented Generation (RAG), the technique powering AI-driven applications like ChatGPT. Generating high-quality embeddings and storing vectors natively within the database could make MongoDB a critical player in AI-driven application development.
But skeptics argue that Voyage AI is just a feature, not a transformative acquisition. AI practitioners know that embeddings alone don’t solve the full challenge of AI search and retrieval—efficient indexing, recall optimization, and cost-effective scaling still require robust vector database capabilities.
Some argue that MongoDB still lags behind dedicated vector databases in core retrieval optimizations. However, recent updates aim to bridge the gap. In December 2024, MongoDB introduced quantization, a feature that reduces vector storage size both on disk and in memory. Additionally, the new dedicated BSON type for vectors further optimizes storage efficiency.
MongoDB’s Future: More Acquisitions Incoming?
One thing is clear: MongoDB is not done yet. This acquisition hints at a larger AI-first database strategy, and more acquisitions are likely. Given its aggressive push into AI-powered search, it wouldn’t be surprising if MongoDB snaps up a dedicated vector DB player to complete the puzzle.