Introduction
The demand-supply landscape is evolving rapidly, driven by globalization, heightened customer expectations, and market volatility. Traditional approaches to supply chain management often struggle to meet the agility, accuracy, and responsiveness required in this dynamic environment. Enter artificial intelligence (AI) as a crucial solution. AI offers advanced data analysis capabilities, processing vast amounts of structured and unstructured data to generate accurate demand forecasts, optimize inventory levels, and streamline resource allocation. AI-driven optimization algorithms enhance various aspects of the supply chain, such as inventory management and transportation routes. Additionally, AI enables risk identification and mitigation by analyzing diverse data sources. By enhancing visibility and transparency, AI empowers organizations to collaborate effectively, improve communication, and achieve greater customer satisfaction. Embracing AI in supply chain management is not only essential for staying ahead, but it is also a strategic imperative to unlock opportunities, mitigate risks, and achieve sustainable growth in today’s rapidly evolving business environment.
AI Applications in Demand-Supply Planning
Algorithms and machine learning techniques have unlocked unprecedented possibilities. AI empowers organizations to tackle complex challenges and make informed decisions in real time. One notable application is demand forecasting, where AI algorithms analyze historical data, market trends, and external factors to provide accurate predictions. These advanced predictive models identify patterns, seasonality, and consumer preferences, enabling organizations to optimize production and inventory levels. Another area where AI excels is inventory optimization. AI-driven inventory management systems leverage real-time data, demand patterns, and supply constraints to optimize inventory levels, reducing costs, improving service levels, and minimizing wastage. Additionally, AI technologies aid in supply chain risk management by analyzing supply chain data and external factors to identify potential risks and disruptions. This proactive approach enhances resilience, ensures continuity, and minimizes the impact of unforeseen events.
The Positive Impacts of AI in Demand-Supply Planning
AI algorithms leverage data-driven insights to improve demand forecasting accuracy, resulting in reduced forecast errors and improved decision-making capabilities. With the ability to analyze vast amounts of real-time data, AI systems enable organizations to make quicker and more informed decisions, ensuring optimal inventory levels, production schedules, and order fulfillment. Moreover, AI-driven optimization algorithms identify opportunities to streamline operations, reduce waste, and maximize resource utilization, leading to cost savings and increased operational efficiency. Demand-supply synchronization is also significantly enhanced through AI, allowing organizations to align supply capabilities with demand fluctuations, resulting in improved responsiveness, reduced stockouts, and enhanced customer satisfaction.
Unveiling the Challenges and Potential Drawbacks of AI in Demand-Supply Planning
But amidst the immense potential, it is essential to acknowledge the challenges and potential drawbacks associated with AI implementation. One significant challenge lies in data quality and integration. AI models heavily rely on high-quality and integrated data from various sources. Ensuring data accuracy, consistency, and compatibility across different systems and stakeholders can be a complex task that requires careful attention. Another challenge is the need for a skilled workforce capable of understanding and effectively utilizing AI capabilities. Organizations may face difficulties in upskilling existing employees and attracting AI talent, potentially leading to workforce disruptions. The integration of AI in demand-supply operations not only revolutionizes the efficiency and optimization of supply chains but also has a significant impact on job roles within this domain. AI technologies automate routine tasks, allowing for a reduction in manual labor and streamlining operational processes. This shift in the workforce requires a transformation of skill requirements, where employees need to possess a combination of technical expertise in AI and data analytics along with domain knowledge in supply chain management. rates new job opportunities related to AI implementation, maintenance, and oversight. This transition leads to a redistribution of job responsibilities, emphasizing the importance of human-AI collaboration. Additionally, the adoption of AI raises ethical concerns regarding data privacy, algorithmic bias, and the potential impact on human labor. Organizations must ensure transparency, fairness, and ethical use of AI technologies to avoid unintended consequences and discriminatory outcomes.
Mitigation Strategies and Recommendations for Successful AI Implementation
To address these challenges and mitigate potential risks, organizations can implement various strategies and recommendations for successful AI implementation in demand-supply operations. Firstly, developing guidelines and frameworks that promote the ethical use of AI, algorithmic transparency, and fairness is crucial. Organizations should invest in upskilling and reskilling programs to enable employees to adapt to the changing demands of an AI-driven landscape. Fostering collaboration between AI systems and human workers is also essential, leveraging the strengths of both while ensuring that humans retain decision-making authority and oversight. Establishing robust data management practices, including data governance frameworks and addressing data integration challenges, is crucial for effective AI implementation. As AI redefines job roles, new positions emerge that focus on managing and optimizing AI-driven systems, interpreting insights generated by AI algorithms, and making strategic decisions based on AI-driven recommendations.
Conclusion
AI has the potential to revolutionize demand-supply planning, offering enhanced accuracy, real-time decision-making, and optimal resource utilization. While challenges and potential drawbacks exist, organizations can overcome them by implementing responsible AI frameworks, investing in workforce adaptation, and ensuring robust data management practices. By embracing AI as a transformative force, organizations can unlock new realms of success in demand-supply operations and seize a competitive advantage in the ever-evolving business landscape.
This article is written by a member of the AIM Leaders Council. AIM Leaders Council is an invitation-only forum of senior executives in the Data Science and Analytics industry. To check if you are eligible for a membership, please fill out the form here.
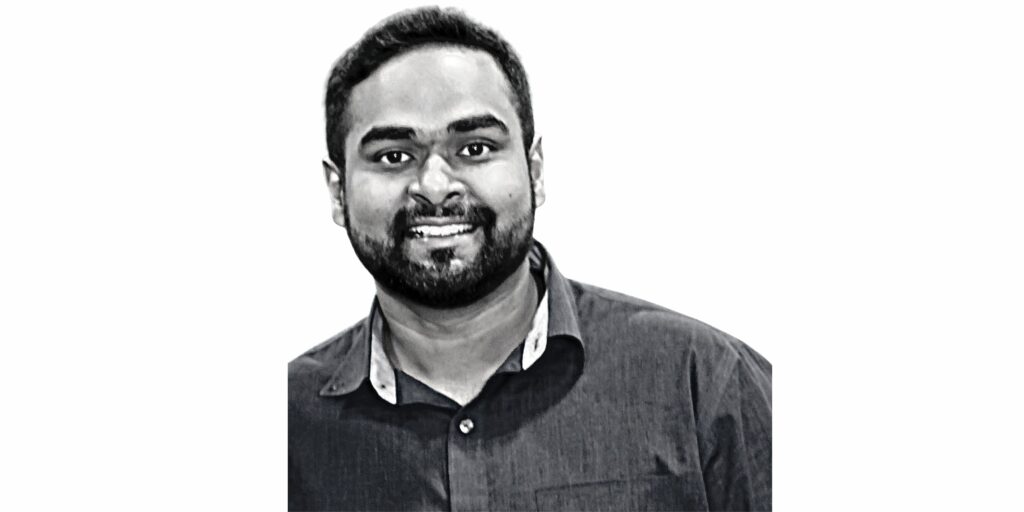
Ruble heads the Centre of Excellence for Data Science, Analytics and Consulting at eClerx. In the last 4+ years, Ruble has built this team grounds up and today drives growth for eClerx by building successful client partnerships in Data Science.