Spot AI, a company that has grown into a key player in the field of Video AI for physical environments, announced today the launch of Iris, its latest product that allows enterprises to build their own custom video AI agents through natural conversation. Touted as the world’s first universal video AI agent builder, Iris is designed for industries such as manufacturing, logistics, retail, construction, and healthcare. The announcement marks a significant expansion of Spot AI’s platform, following its Fall 2024 release of prebuilt Video AI Agents for safety, security, and operations.
Iris enables users to create agents tailored to their specific operational needs without any prior experience in coding or machine learning. Rather than relying on teams of data scientists or weeks of model training, business leaders can now instruct the system through a simple conversational interface. According to Spot AI, what previously required eight weeks of development and thousands of annotated images can now be accomplished in just eight minutes and twenty training images.
“Iris transforms how we interact with video data through natural conversation,” said Rish Gupta, CEO and Co-Founder of Spot AI. “What ChatGPT did for text, Iris does for video-making powerful AI capabilities accessible without requiring technical expertise. Iris will help anyone build an AI agent that watches videos and acts on their behalf–from retailers and hospitals to the industrial heart of America.”
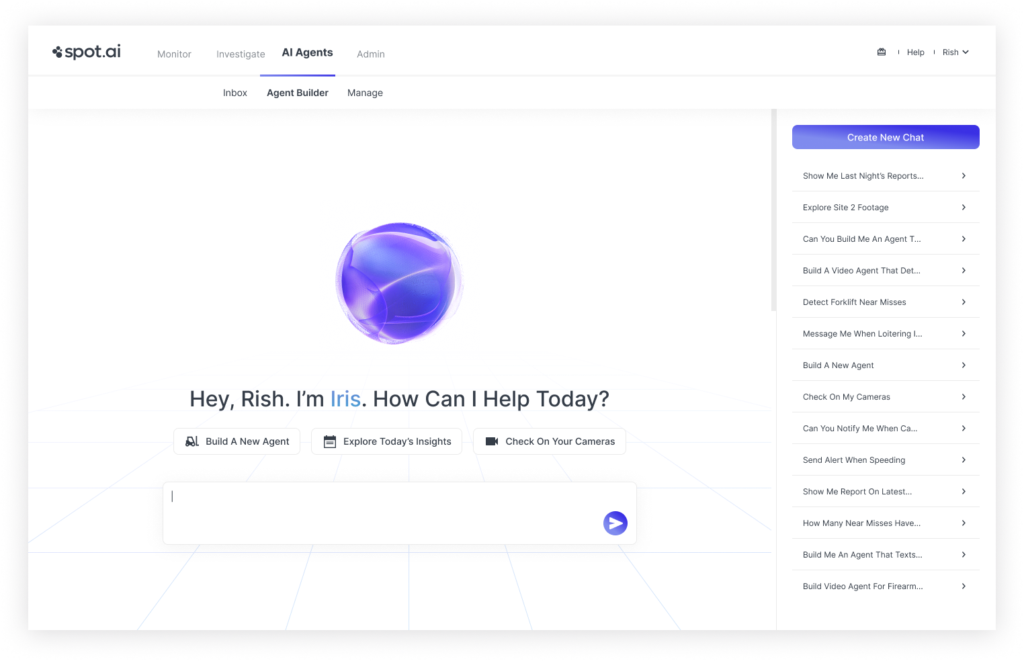
The system builds on a core principle that for AI to move beyond digital interfaces and into real-world operations, it must be able to see. With over 100 million enterprise cameras deployed across the United States, Spot AI sees a significant opportunity in turning these passive devices into intelligent agents that can observe, interpret, and respond to activity in real-time. The company’s earlier agents, launched in late 2024, were pre-trained to address a range of common workplace scenarios, including reducing injuries, minimizing security risks, and preventing operational inefficiencies.
Iris extends this functionality by giving organizations the tools to define their own use cases. It allows users to teach the system with positive and negative reinforcement to fine-tune accuracy based on the particular requirements of a business environment. Beyond analysis, Iris can also trigger physical-world actions such as stopping machines, locking doors, or activating tasks via integrations, depending on the situation detected in the video footage.
Gupta emphasized that the simplicity of building with Iris is what sets it apart. He noted that before Iris, building specialized video detection systems required highly specialized teams, advanced degrees, and weeks of effort. Now, those same capabilities are being offered to decision-makers directly, removing the dependency on dedicated AI engineering teams.
Spot AI highlighted a number of examples of how Iris is already being used to address industry-specific challenges. In manufacturing, companies are training agents to detect product backups on production lines or identify leaks, with automated responses triggered based on the severity of the issue. In warehousing environments, Iris can identify when pallets or boxes are stacked unsafely, allowing teams to intervene before accidents occur. In retail, store managers are using Iris to monitor shelf stock levels and receive timely alerts when specific items run low. Hospitals are using the platform to distinguish between patients and healthcare workers in scrubs, improving traffic flow and reducing the risk of cross-contamination or confusion. Security teams are deploying agents that detect theft tools like bolt cutters in parking lots, helping them respond to new and evolving threats. Other organizations are using the system to ensure compliance with safety protocols, such as identifying whether specialized roles are wearing the appropriate gear.
According to Spot AI, the agents continuously monitor critical areas, helping reduce workplace incidents and operational inefficiencies with minimal manual oversight. While the earlier versions of the platform were effective in solving widespread challenges, Iris is meant to address the long tail of enterprise-specific problems where prebuilt solutions often fall short.
The company has also prioritized ease of deployment. Spot AI’s platform is compatible with existing IP camera infrastructure, which means businesses can adopt the technology without undergoing costly overhauls. Video processing is handled on-site through Spot AI’s own hardware, with only essential data sent to the cloud. This setup reduces latency, improves speed, and ensures that sensitive information remains secure—an important factor for industries with strict data policies, such as healthcare and education.
As of 2024, Spot AI has become a trusted video AI provider to more than 1,000 organizations, processing a volume of video data that, according to the company, rivals the daily upload rates of YouTube. Across sectors, the platform has been adopted for a variety of tasks: automotive manufacturers use it to monitor assembly lines for both product quality and worker safety, school administrators rely on it to manage student safety in real-time, and retail chains have used it to reduce shrinkage and identify service issues that impact customer experience.
The launch of Iris will be officially presented by CEO Rish Gupta during a media roundtable at Google Cloud Next on April 9. The timing of the announcement reflects Spot AI’s growing visibility within enterprise AI conversations, particularly those focused on bridging the divide between digital tools and real-world operations. While the technical capabilities of the platform are extensive, Spot AI’s leadership is betting that its simplicity will be the true differentiator especially in industries where AI has often been seen as out of reach due to cost, complexity, or the need for specialized expertise.
With Iris, Spot AI is shifting the control of AI agents into the hands of the people closest to the work who understand the problems but have never had the tools to solve them at scale.