Businesses across industries are embracing various AI-based solutions – be it for automating tasks, gaining insights, or for data-driven decision-making. Amidst this transformative wave, it’s evident that AI integration requires a comprehensive strategy that extends beyond algorithmic excellence. The success of enterprise-scale AI projects can depend on more than just the quality of algorithms and models- it can heavily depend on the operational excellence (OE) it achieves during the implementation process.
What is Operational Excellence?
Operational excellence aims to continuously measure and improve the effectiveness of deliverables, while also assessing the efficiency of the development process. It involves understanding business goals, identifying key performance indicators (KPIs), and driving strong engineering practices to deliver high-quality and reliable outcomes, efficiently. Operational excellence in software engineering has evolved with well-established processes and practices such as Agile methodologies, DevOps, modular designs, and automated deployments. However, in the realm of data science, the application of OE is nascent; it is a relatively modern approach that is gaining momentum as the outcome and effectiveness of AI solutions depend heavily on its measures.
Data science projects involve various stages, including data collection, curation, model training, validation, deployment, and maintenance. Each stage requires continuous improvement and adapting to evolving data and dynamic business requirements. While organizations can measure the effectiveness of data science initiatives by evaluating business impact, model accuracy metrics, and performance metrics, it is crucial to also measure the efficiency of data science models in the development process. By analyzing the efficiency of data preparation and management, including aspects like data quality, completeness, consistency, timeliness, duplication, bias, and availability, organizations can optimize the data processing pipeline. Additionally, metrics related to model training time, validation coverage, version control, deployment time, inference speed, and proactive monitoring allow for the continuous improvement and optimization of the data science workflow.
Data Preparation & Management
Effective data utilization, a foundational element in AI, is intricately tied to proficient Data Preparation and Management practices. Assessing data quality, completeness, consistency, timeliness, and mitigating biases is crucial to underpinning trustworthy and precise AI results. These metrics collectively form the bedrock of successful AI implementation, enabling organizations to harness the full potential of their data resources for informed decision-making and innovation.
- Availability of Data and Quality: Assessing the accessibility, storage, and governance of data is essential to ensure that it can be effectively used for AI initiatives. At the same time, evaluating the data quality for data integrity, consistency, and relevance can impact the accuracy and reliability of AI solutions.
- Data Consistency & Completeness: Ensuring data consistency involves checking for uniformity and coherence across different data sources. It helps identify any discrepancies or inconsistencies in the data that could impact the accuracy and reliability of the AI models. Data Completeness assesses the extent to which the dataset includes all the required fields and values. It ensures that the data is comprehensive and contains sufficient information for analysis and modeling.
- Data Timeliness: Evaluating whether the data is up-to-date and relevant for the AI solutions ensures that the data reflects the most recent information and avoids any potential issues caused by outdated or irrelevant data.
- Data Duplication: Detecting and quantifying duplicate data entries and records avoids redundancy and maintains data accuracy.
- Bias in Dataset: Finally, addressing bias in the dataset ensures fairness and prevents any discriminatory outcomes in AI models. This crucial metric involves the elimination of biases based on factors such as demographics, representation, or sampling methods.
Model Training & Deployment
In the dynamic landscape of AI integration, a strong foundation in model training and deployment sets the stage for data-driven success. Delving into the realm of model training and deployment, essential metrics come to light. These metrics include model training time, validation coverage, model version control, and deployment speed. Each metric plays a pivotal role in not only ensuring the robust performance of AI models but also streamlining their journey from development to real-world application. Optimizing these aspects can enable organizations to unlock the true potential of AI and make informed decisions swiftly.
- Model Training Time: Evaluating the efficiency of a training process and the duration needed to train a data science model is essential. By optimizing resources and utilizing parallel computing or distributed systems, the model training phase can be accelerated.
- Validation Coverage: Measuring the extent to which the model is validated across different scenarios or datasets ensures that the model performs well and maintains accuracy across various inputs, providing a comprehensive assessment of its reliability. By achieving high validation coverage, organizations can have confidence in the model’s generalizability and effectiveness.
- Model Version Control: Managing and tracking different versions of the model throughout its lifecycle enables organizations to maintain a clear record of changes, improvements, and updates made to the model over time. Proper version control ensures traceability and facilitates collaboration among data scientists and stakeholders.
- Model Deployment Time and Inference Speed: These metrics focus on the efficiency of deploying the trained model into a production environment and the speed at which the model can make accurate predictions or inferences. Minimizing the time required for model deployment allows for faster implementation and responsiveness in real-time applications. Similarly, optimizing the inference speed ensures that predictions or results are delivered swiftly, enhancing the user experience and enabling timely decision-making.
Post-Model Deployment
Finally, as organizations operationalize AI models, the phase of post-model deployment becomes equally critical. This involves continuous monitoring of model performance and timely resolution of any issues that arise, ensuring smooth functioning and optimal outcomes. This broadly includes two metrics:
- Proactive Monitoring: Monitoring the performance of deployed data science models, identifying any deviations or issues such as model drift, and taking proactive measures to address them in a timely manner.
- Resolution Time: Measuring the speed at which identified issues and anomalies are resolved and incidents are effectively managed for smooth functioning and optimal performance of the data science models.
Building AI-based solutions can help businesses achieve strategic objectives and gain a competitive edge. In fact, according to a report by PWC, 54% of executives surveyed claim that their companies’ productivity has already grown because of AI technologies. Nearly half of the respondents in a Business Transformation & Operational Excellence (BTOE) poll reported having a formal enterprise-wide operational excellence program (45%), and 54% said they thought more people were becoming aware of operational excellence approaches.
In conclusion, the adoption of AI-based solutions necessitates the incorporation of operational excellence principles. As businesses navigate the complexities of AI implementation, focusing on both technical and operational aspects is crucial for achieving successful outcomes. By following best practices, leveraging appropriate metrics, and continuously improving processes, organizations can harness the power of AI while maintaining operational excellence.
This article is written by a member of the AIM Leaders Council. AIM Leaders Council is an invitation-only forum of senior executives in the Data Science and Analytics industry. To check if you are eligible for a membership, please fill out the form here.
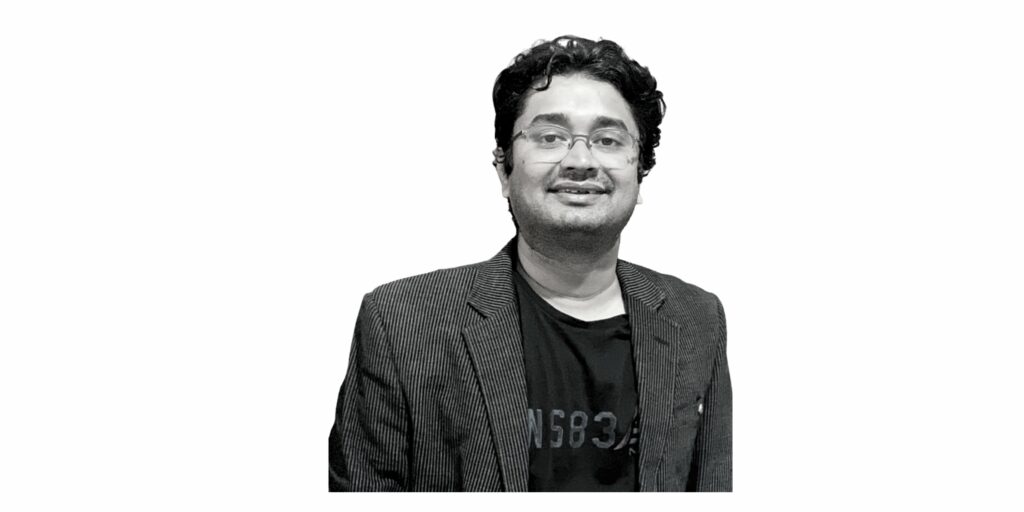
Swaroop has 17+ years of rich experience in IP based video cameras, surveillance and computer vision technology building & managing enterprise scale video solutions. He has been a passionate evangelist of video analytics technology and has 2 patents in this domain. He is involved in incubating & transforming retail organisations using AI/ML computer vision technology. He is currently leading the computer vision platform at Lowe’s as Director – Data Science.