Today, we live in an “Age of With” where it is not a survival competition against AI but rather a collaboration to elevate human endeavors. AI has generated considerable buzz in the pharmaceutical sector over the past years, and the recent surge in interest around generative AI (ChatGPT) is intensifying discussions even further. Presently, there exists a divergence in perspectives, with some expressing enthusiasm for its potential, while others exhibit caution—this divergence is normal and quite expected. After all, AI have been supporting various sectors in the pharmaceutical world differently.
AI Transforming the Pharmaceutical Industry
The pharmaceutical industry is witnessing the steady integration of artificial intelligence (AI) across various domains, including drug development, operations, and marketing. Projections indicate that the AI market in the pharmaceutical sector is set to reach $10 billion by 2024. This encompasses a spectrum of AI applications, ranging from accelerated drug discovery and design, to supply chain management optimization to revolutionizing personalized medicine.
AI’s impact on the biopharmaceutical sector is multifaceted:
Drug Discovery and Design
AI plays a pivotal role in drug discovery and design, from creating new molecules to identifying novel biological targets. Its involvement spans target identification and validation, target-based and phenotypic drug discovery, drug repurposing, and biomarker identification. Leveraging AI during drug trials can significantly reduce timelines to bring essential and innovative medicine to a much needing patient population, translating into improving and extending patient’s lives. This also has a significant impact on overall drug discovery costs, which results in lower retail drug prices that improve patient access to treatment. A great example is how Pfizer was able to expedite early stages of drug research by using the IBM Watson for Drug Discovery initiative, where it leverages the use of natural language processing (NLP) techniques to analyze vast volumes of scientific literature, clinical trials data, and research papers and extract crucial insights, and generate hypotheses for potential drug candidates.
Identifying Clinical Trial Candidates
According to research conducted by Cognizant, approximately 80% of clinical trials struggle to adhere to their enrollment schedules. Moreover, one out of every three Phase III clinical trials are discontinued due to challenges related to participant recruitment. can be a great asset in tackling such challenges with its ability to process vast amounts of data, usually in free text form, like doctors’ notes and intake documents and analyze specific and detailed inclusion and exclusion criterions. This significantly accelerates identification of clinical trial candidate patients, which in turn, significantly accelerate research timelines and eventually patient access to live saving drugs.
Predictive Biomarkers
AI employs biomarker models trained on extensive datasets to develop predictive biomarkers. These biomarkers identify potential responders to molecular targeted therapies before human trials, presenting a valuable strategy for pharmaceutical companies to repurpose drugs with reduced risk and R&D expenditure.
Manufacturing Process Enhancement
AI offers an array of opportunities to enhance processes in development and production. It contributes to quality control, shortens design times, minimizes material wastage, improves production efficiency, facilitates predictive maintenance, and more. For instance, processes reliant on human intervention for data input or management can be streamlined through computer numerical control (CNC) driven by AI machine learning algorithms. This not only ensures precise task execution but also identifies areas for process optimization, resulting in reduced waste and increased production speed while maintaining product quality.
Personalized Medicine
AI significantly reduces the trial-and-error approach in drug interventions by matching drugs to individual patients based on predictive modeling. Machine learning models predict patient responses to potential drug treatments by inferring relationships among factors influencing outcomes, such as drug absorption, compound distribution, metabolism, and predictive biomarkers. This personalizes and tailors the treatment journey for the patient’s needs.
Pharmaceutical companies are increasingly turning to AI to expedite the introduction of new discoveries to the market and enhance patients’ lives. The recent surge in AI implementation is showing no signs of slowing down. Recent research indicates that approximately 50% of global healthcare companies are poised to adopt AI strategies and integrate the technology into their operations by 2025.
However, there is a reluctance to embrace “black box” AI systems, particularly deep learning models and generative AI models, as they often provide groundbreaking results without clear explanations comprehensible to humans. Nevertheless, there is a growing interest in developing self-explanatory AI models, commonly known as “explainable AI”
AI and machine learning continue to drive innovation in drug discovery, manufacturing, and personalized treatments. As these AI tools become more accessible and interpretable over the years, they will become part of the natural process within the pharmaceutical industry.
References:
Smith, A. R., & Brown, E. N. (2018). Rethinking clinical trials: The role of machine learning in the COVID-19 era. Seizure: European Journal of Epilepsy, 83, 127-129.
Topol, E. J. (2019). High-performance medicine: the convergence of human and artificial intelligence. Nature Medicine, 25(1), 44-56.
Angermueller, C., Pärnamaa, T., Parts, L., & Stegle, O. (2016). Deep learning for computational biology. Molecular systems biology, 12(7), 878.
Rajkomar, A., Dean, J., & Kohane, I. (2019). Machine learning in medicine. New England Journal of Medicine, 380(14), 1347-1358.
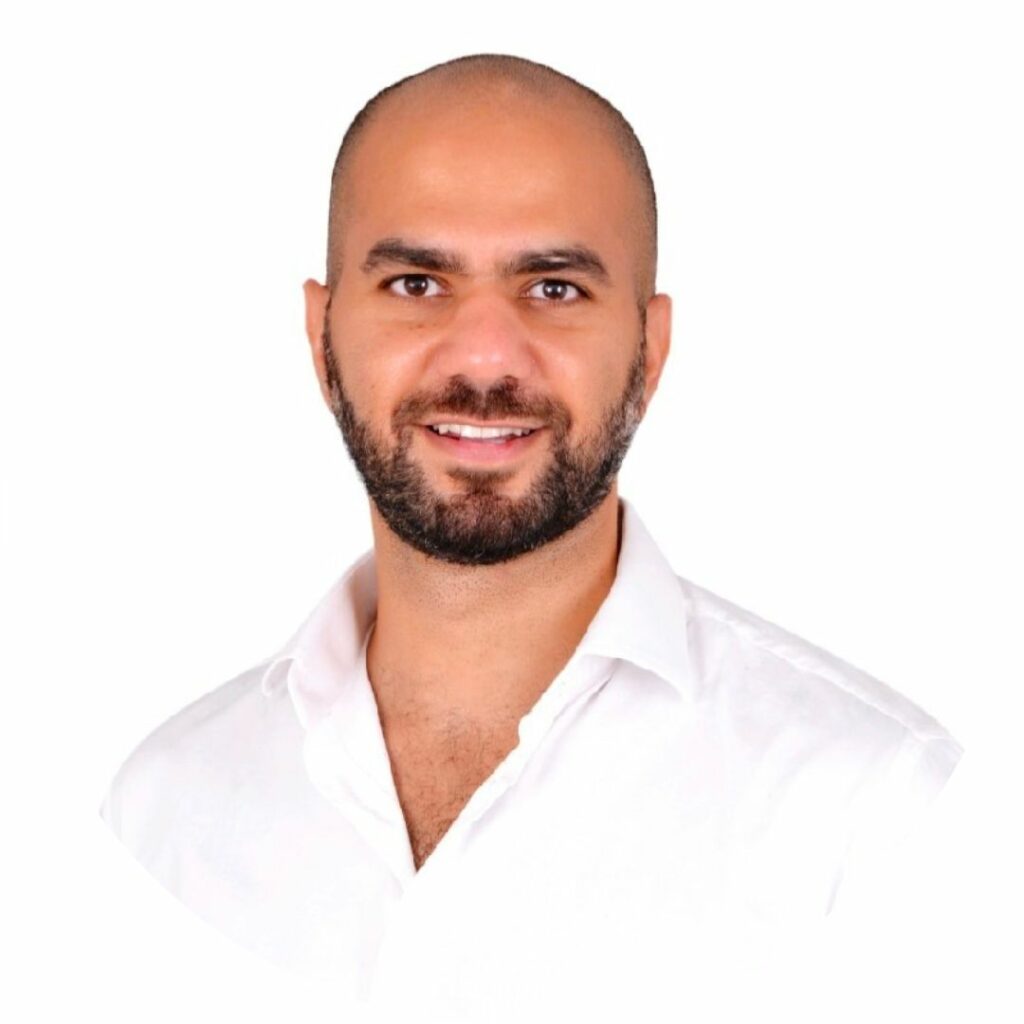
Moutaz is an accomplished Global Associate Director for Reporting and Analytics at Novartis, with a decade of expertise in healthcare analytics and commercial strategy. He leads high-performing teams, manages multi-million-dollar portfolios, and drives data-driven decisions for remarkable growth and innovative solutions in healthcare.