The gap between AI’s promise and its practical implementation remains frustratingly wide for many companies. While headlines celebrate breakthrough technologies and billion-dollar valuations, the reality on the ground often involves failed pilots, underwhelming results, and the sobering recognition that simply adding AI features to existing processes rarely delivers transformative value.
This discussion brings together industry leaders who have navigated these challenges firsthand. They’ve moved beyond the hype to implement AI-first strategies that deliver measurable results. The panel features Arun Shastry, who manages the Data Analytics Delivery Organization at Marsh McLennan, Doug Jauregui, who is Sales Engineering Manager at Fivetran and Arjun Srinivasan, who heads the Data Science and Enterprise AI for WESCO.
Transforming Sales Operations with Embedded AI
In enterprise environments, AI integration often begins where it delivers the most immediate impact. Consider the modern CRM landscape, where platforms like Dynamics 365, HubSpot, and Salesforce now embed AI capabilities by default. For organizations with substantial sales forces, this presents unprecedented opportunities for optimization.
In industrial distribution, where margins are typically low and volume drives profitability, AI-enabled lead generation and scoring has proven transformative. Traditional approaches required sales teams to spend over 20% of their time on lead assessment and qualification activities. By leveraging AI systems that analyze historical data, market indicators, economic trends, and competitive intelligence, organizations can automate these processes entirely.
The results speak for themselves. Sales teams redirect their focus from administrative tasks to actual selling, increasing deal velocity and expanding opportunity pipelines. This shift requires proper data governance and quality management as foundational elements, but when implemented correctly, it directly impacts top-line revenue growth.
Modernizing Legacy Systems for AI Readiness
The challenge of legacy system integration represents a critical hurdle for many organizations pursuing AI-first strategies. However, the solution often involves leveraging existing data governance frameworks rather than rebuilding from scratch.
Organizations that have invested in analytics-ready datasets possess a significant advantage. The same governance, security, and access controls that protect traditional analytics can be extended to AI implementations. The key lies in the additional step of vectorization—transforming existing data into AI-ready formats that can be consumed by language models and other AI systems.
A compelling example comes from the manufacturing sector, where a cement manufacturer successfully implemented an AI-first approach using Microsoft’s Copilot ecosystem. By maintaining their existing governance structure while vectorizing their data, they enabled organization-wide access to AI-generated insights and documentation. Employees can now generate ad hoc visualizations and derive intelligence from their comprehensive data ecosystem, including ERP systems, relational databases, and application data all while maintaining appropriate access controls based on user permissions.
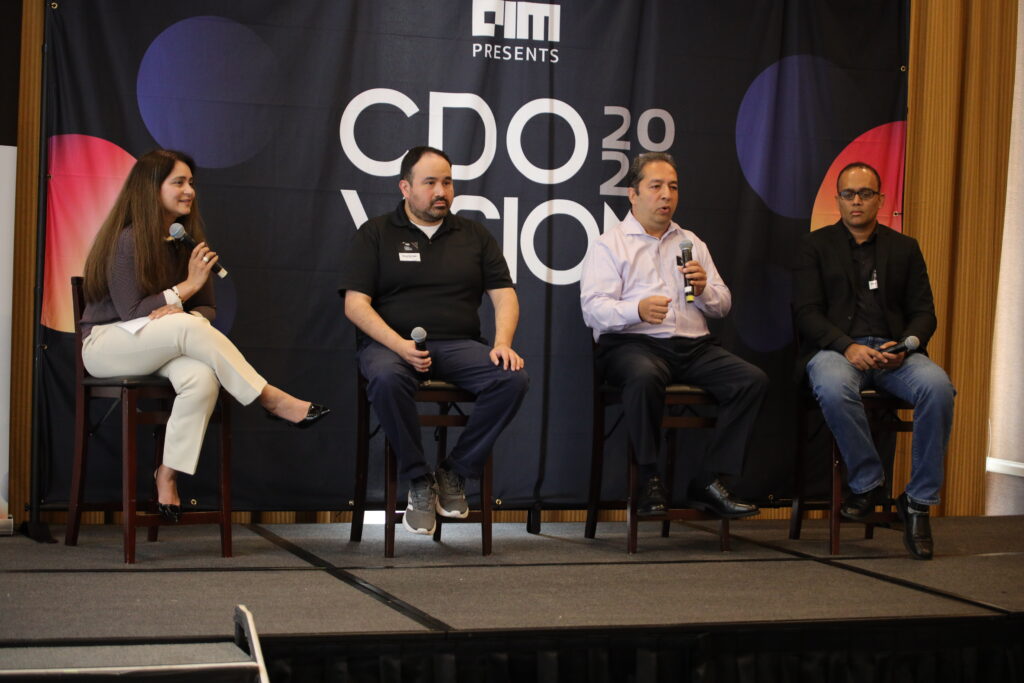
Moving Beyond Bolt-On Solutions
The temptation to simply add AI features to existing products often leads to suboptimal outcomes. As one industry expert noted, “Adding AI to a traditional application is like putting a Ferrari sticker on a Prius it’s still not going to race well with a Ferrari.”
True competitive advantage requires starting with an AI mindset from the ground up. This means beginning with user experience design and working backwards to determine how AI can personalize interactions, predict user behavior, and automate routine processes. While bolt-on AI solutions might have impressed customers two years ago, today’s market demands more sophisticated, integrated experiences.
Modern customers expect applications that demonstrate deep understanding of their needs, can anticipate their next actions, and provide optimized experiences at every touchpoint. Meeting these expectations requires robust customer analytics, predictive behavior modeling, and proactive functionality that stays two steps ahead of user needs.
The Foundation of AI Readiness
The progression from AI-ready to AI-first cannot be rushed or shortcut. Organizations must establish solid foundations in several key areas before expecting successful AI implementations.
Data quality and integrity remain paramount. Whether building custom models with internal data science teams or purchasing AI solutions, the underlying data determines the quality of AI outputs. This necessitates dedicated teams focused on data governance, quality assurance, and infrastructure readiness.
For organizations adopting third-party AI solutions, robust monitoring and observability tools become essential. When AI systems make decisions, human oversight and root cause analysis capabilities ensure accountability and continuous improvement. The goal is not full automation but rather augmented decision-making where humans remain in the loop.
Grassroots Innovation and Organizational Change
Sometimes the most effective AI implementations begin with individual initiative rather than top-down mandates. Consider the example of a sales professional who needed better access to historical ticketing data to improve customer interactions. By vectorizing existing Jira and Zendesk data, they created a system that could instantly provide relevant solutions and resolutions for specific customer issues.
This grassroots approach proved so valuable that it spread throughout the organization. Team members began requesting automated documentation generation for prospect follow-ups, leading to broader product development initiatives. This bottom-up innovation often proves more sustainable than enterprise-wide AI mandates because it addresses real user needs and demonstrates immediate value.
Business-First, Technology-Second Approach
A critical mistake many organizations make involves starting with technology rather than business objectives. Success requires beginning with clear business use cases and well-defined problem statements. Only after establishing these foundations should organizations move to solution design, AI implementation, and customer experience optimization.
Organizations that jump directly to technical implementation face approximately a 50% success rate. Those that begin with business strategy and work systematically through use case definition, solution architecture, and AI integration achieve significantly better outcomes.
The Evolving Executive Landscape
The executive landscape around AI has transformed dramatically over the past 18 months. The emergence of Chief AI Officer roles in publicly traded companies signals the strategic importance of AI initiatives. Today, virtually every major organization either has dedicated AI practices or has integrated AI strategy into their existing technology leadership structure.
This shift has been driven by multiple converging forces. Consumer expectations for AI-enhanced experiences create market pressure. Stakeholder demands for competitive positioning drive investment decisions. Government initiatives and policies further emphasize the strategic importance of AI capabilities.
Recent political developments have accelerated these trends, with increased investment flowing into AI initiatives regardless of organizational political perspectives. Companies recognize that AI investment has become essential for competitive positioning and stakeholder confidence.
The Double-Edged Sword of AI Investment
The current environment presents both unprecedented opportunities and significant risks for AI practitioners. The positive aspect is clear: funding for AI initiatives is readily available. Board-level conversations consistently include AI strategy discussions, and senior leadership actively seeks AI investment opportunities.
However, this enthusiasm creates elevated expectations. Organizations investing significant resources in AI initiatives expect measurable returns. A million-dollar AI investment typically requires demonstration of five million dollars in value creation within two to three years. This could manifest as new revenue opportunities, customer engagement improvements, operational automation, or cost reduction.
The challenge lies in selecting appropriate use cases and delivering measurable value. With organizational leadership willing to fund AI initiatives, practitioners must focus intensively on identifying high-impact applications and establishing clear success metrics.
Understanding AI-Ready vs. Analytics-Ready Data
The distinction between analytics-ready and AI-ready data often causes confusion among organizations beginning their AI journey. The fundamental difference lies in data structure and accessibility rather than governance or security requirements.
AI-ready data typically involves vectorization transforming traditional data formats into vector representations that can be consumed by modern AI systems. Most contemporary databases now support vector storage capabilities, allowing organizations to maintain their existing data repositories while adding AI-specific schemas.
This approach enables organizations to leverage their existing medallion architectures and data governance frameworks while extending capabilities to support generative AI applications. The same data that powers traditional analytics can be transformed and vectorized to enable AI-driven insights without compromising existing security or access controls.
Socializing AI Strategy Across Organizations
Successfully implementing AI-first strategies requires organization-wide alignment and understanding. This involves education, demonstration of value, and gradual expansion of AI capabilities across different business functions.
The most effective approaches combine executive support with grassroots innovation. While board-level commitment provides necessary resources and strategic direction, practical implementation often succeeds through small-scale pilots that demonstrate tangible value to end users.
Conclusion
The transition to AI-first operations represents both a significant opportunity and a complex challenge for modern organizations. Success requires careful attention to foundational elements like data quality and governance, strategic thinking about use case selection, and systematic approaches to implementation.
Organizations that begin with clear business objectives, invest in proper data foundations, and maintain focus on measurable value creation position themselves for long-term success in the AI-driven economy. The current environment provides unprecedented support for AI initiatives, but this opportunity comes with correspondingly high expectations for demonstrable results.